This Machine Learning Research from Stanford and Microsoft Advances the Understanding of Generalization in Diffusion Models
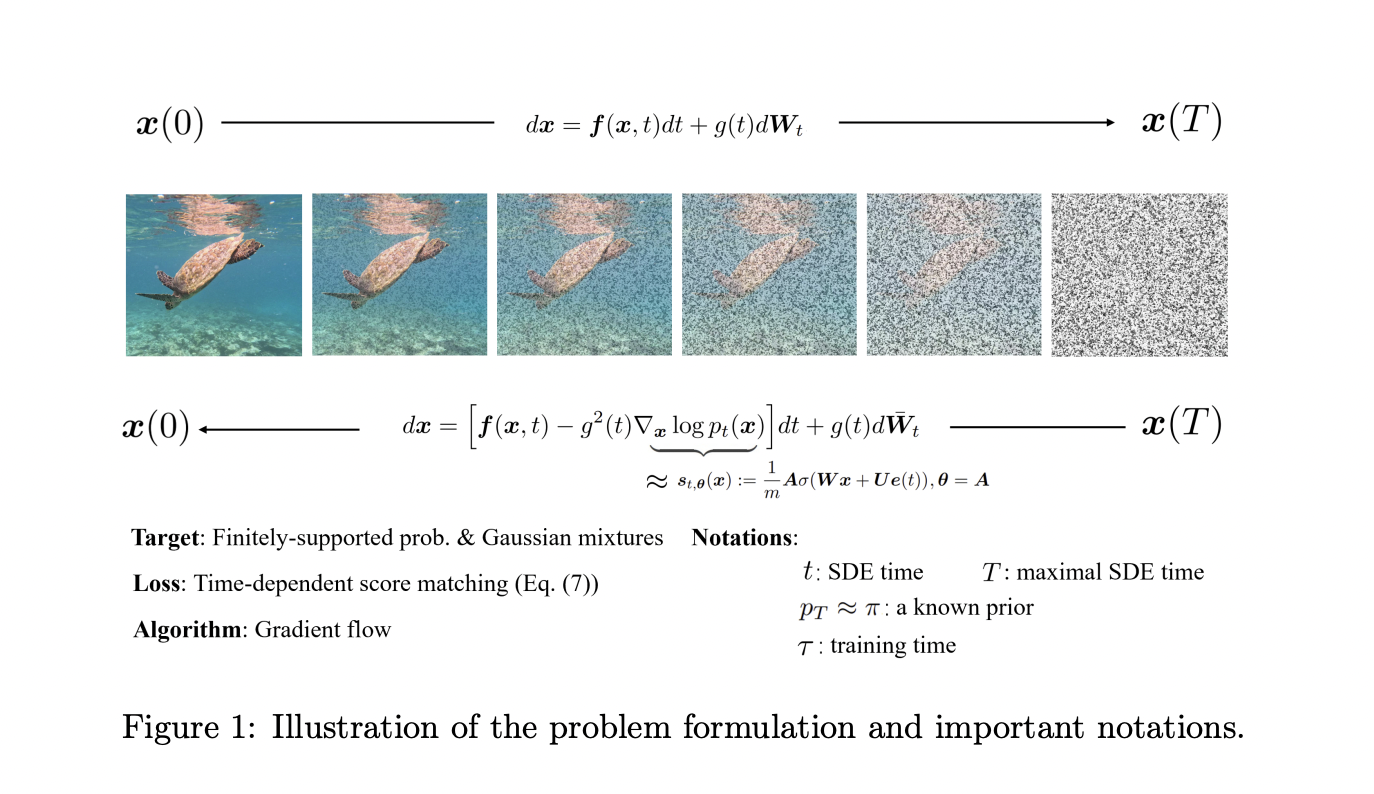
[ad_1]
Diffusion models are at the forefront of generative model research. These models, essential in replicating complex data distributions, have shown remarkable success in various applications, notably in generating intricate and realistic images. They establish a stochastic process that progressively adds noise to data, followed by a learned reversal of this process to create new data instances.
A critical challenge is the ability of models to generalize beyond their training datasets. For diffusion models, this aspect is particularly crucial. Despite their proven empirical prowess in synthesizing data that closely mirrors real-world distributions, the theoretical understanding of their generalization abilities has yet to keep pace. This gap in knowledge poses significant challenges, particularly in ensuring the reliability and safety of these models in practical applications.
Current approaches to diffusion models involve a two-stage process. Initially, these models introduce random noises into data in a controlled manner. They also employ a denoising process to reverse this noise addition, thereby enabling the generation of new data samples. While this approach has demonstrated considerable success in practical applications, the theoretical exploration of how and why these models can generalize effectively from seen to unseen data still needs to be developed. Addressing this gap is imperative for a deeper understanding and more reliable application of these models.
The study introduces groundbreaking theoretical insights into the generalization capabilities of diffusion models. Researchers from Stanford University and Microsoft Research Asia propose a novel framework for understanding how these models learn and generalize from training data. This involves establishing theoretical estimates for the generalization gap – measuring how well the model can extend its learning from the training dataset to new, unseen data.
The research adopts a rigorous mathematical approach. The researchers first establish a theoretical framework to estimate the generalization gap in diffusion models. This framework is then applied in two scenarios, one that is independent of the data being modeled and another that considers data-dependent factors as follows:
- In the first scenario, the team demonstrates that diffusion models can achieve a small generalization error, thus evading the curse of dimensionality – a common problem in high-dimensional data spaces. This achievement is particularly notable when the training process is halted early, a technique known as early stopping.
- In the data-dependent scenario, the research extends its analysis to situations where target distributions vary regarding the distances between their modes. This is critical for understanding how changes in data distributions affect the model’s ability to generalize.
Through mathematical formulations and simulations, the researchers confirm that diffusion models can generalize effectively with a polynomially small error rate when appropriately stopped early in their training. This finding mitigates the risks of overfitting in high-dimensional data modeling. The study reveals that in data-dependent scenarios, the generalization capability of these models is adversely impacted by the increasing distances between modes in target distributions. This aspect is crucial for practitioners who rely on these models for data synthesis and generation, as it highlights the importance of considering the underlying data distribution during model training.
In conclusion, this research marks a significant advancement in our understanding of diffusion models, offering several key takeaways:
- It establishes a foundational understanding of the generalization properties of diffusion models.
- The study demonstrates that early stopping during training is crucial for achieving optimal generalization in these models.
- It highlights the negative impact of increased mode distance in target distributions on the model’s generalization capabilities.
- These insights guide the practical application of diffusion models, ensuring their reliable and ethical usage in generating data across various domains.
- The findings are instrumental for future explorations into other variants of diffusion models and their potential applications in AI.
Check out the Paper and Github. All credit for this research goes to the researchers of this project. Also, don’t forget to follow us on Twitter. Join our 36k+ ML SubReddit, 41k+ Facebook Community, Discord Channel, and LinkedIn Group.
If you like our work, you will love our newsletter..
Don’t Forget to join our Telegram Channel
Hello, My name is Adnan Hassan. I am a consulting intern at Marktechpost and soon to be a management trainee at American Express. I am currently pursuing a dual degree at the Indian Institute of Technology, Kharagpur. I am passionate about technology and want to create new products that make a difference.
[ad_2]
Source link